UGAT - Uncertainty Aware Grounded Action Transformation for Traffic Signal Control
First attempt to use Grounded Action Transformation (GAT) to solve the Sim2Real Deployment Issue in the Traffic Signal Control domain. By inferring the real-world dynamics using a `forward model`, the RL agent’s actions are grounded based on reality-based rectification. Furthermore, the researchers propose to apply uncertainty quantification to judge the action’s reliability before taking the grounded action. We also propose a dynamic updating mechanism to maintain the acceptance threshold and guarantee a steady learning process. Paper link
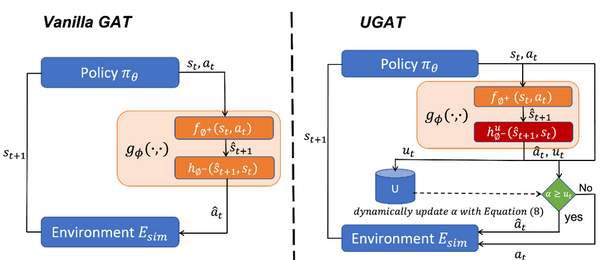
CrowdGAIL
GAIL applied to agent navigation tasks. In this project, Generative Adversarial Imitation Learning is futher explored and inherited from PPO as the policy optimization method, which later justified outperforms the TRPO. LSTM which special designed Sequential DemoBuffer were also introduced to help depicting the sequential feature of a decision maker agent. This work can be beneficial for motion reasoning tasks for autunomous driving scenes. Paper link
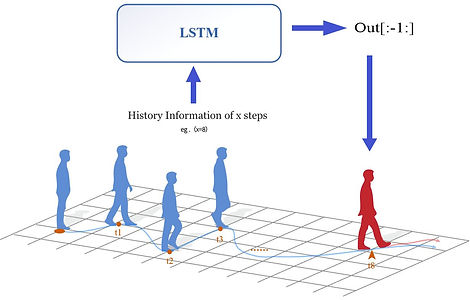.jpeg)
LibSignal
Cross simulator Traffic Signal Control project
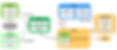
Smart Community
A platform for volunteer activities publishing and procedure sharing, to guarantee the service safety and quality within a certain community
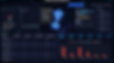